Unlocking the Potential: Data Annotation in Machine Learning
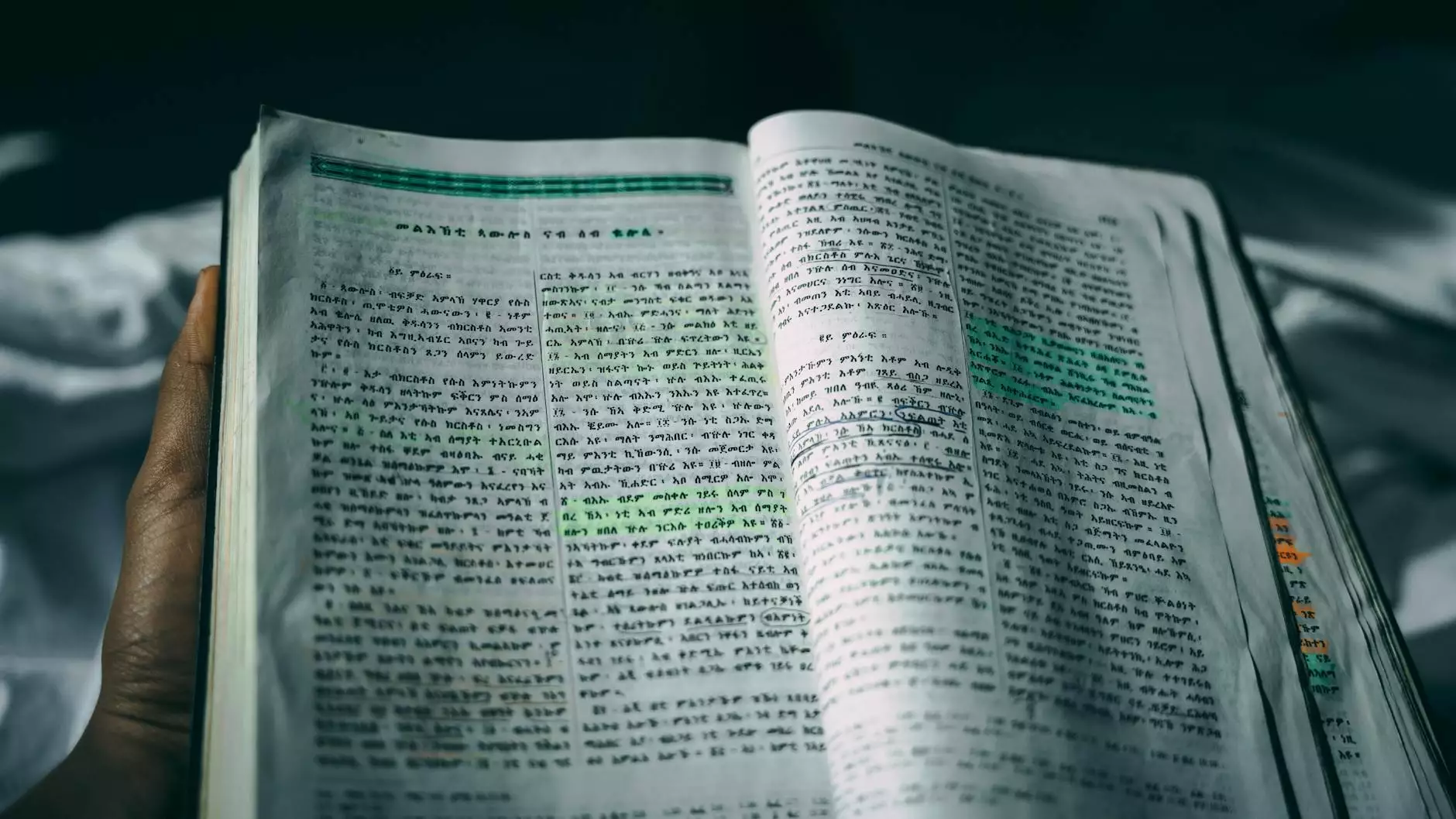
Data annotation machine learning is a critical process that fuels the development of innovative technologies across various industries. In particular, businesses in fields such as Home Services, Keys & Locksmiths can dramatically benefit from effective data annotation practices. As we delve into this topic, we’ll explore the importance of data annotation, its methodologies, and how companies like KeyMakr harness its power to optimize their services.
Understanding Data Annotation
At its core, data annotation involves labeling or tagging data to make it understandable for machine learning models. This process is essential because machine learning algorithms learn from labeled data to make predictions or classifications. Without quality data annotation, the effectiveness of machine learning applications can be severely compromised.
Types of Data Annotation
Data annotation can be categorized into several types, depending on the nature of the data being labeled. Below are some prevalent types:
- Image Annotation: Involves labeling images for use in computer vision applications. This can range from bounding boxes around objects to segmentation masks.
- Text Annotation: This includes tagging parts of speech in texts or identifying sentiment, which is crucial for natural language processing (NLP).
- Audio Annotation: Labeling audio data for speech recognition systems, identifying different speakers, or enhancing sound classification.
- Video Annotation: Tagging sequences in video content, which is vital for training models related to video analysis.
The Role of Data Annotation in Machine Learning
Machine learning thrives on quality data, and data annotation machine learning serves as the bridge between raw data and usable information. Here’s how it plays a significant role:
1. Improved Model Accuracy
Labeled data helps machine learning models understand the nuances of the input data. The more accurately data is annotated, the better the model can learn, which leads to higher prediction accuracy. For instance, a locksmith service using a predictive model for estimating response times can achieve better results with well-annotated historical data.
2. Enhanced User Experience
Data annotation can significantly improve user experience by personalizing services. For example, by analyzing customer feedback through text annotation, businesses can tailor their offerings to meet specific client needs effectively.
3. Scalability of AI Applications
Scalable machine learning applications rely heavily on vast amounts of data. Efficient data annotation processes ensure that businesses can quickly and accurately label new data as they grow, maintaining operational efficiency.
Key Steps in Data Annotation
Implementing an effective data annotation process involves several key steps:
Step 1: Defining Annotation Guidelines
Establishing clear and detailed guidelines is crucial for ensuring consistency and accuracy in annotation. These guidelines should cover aspects such as what data to annotate, how to categorize it, and any specific labeling criteria that should be followed.
Step 2: Choosing the Right Tools
With numerous tools available for data annotation machine learning, selecting the right one can greatly influence the quality of the annotation process. Consider user-friendly interfaces and functionalities that align with your business needs.
Step 3: Training the Annotators
The quality of the annotation is heavily dependent on the annotators' expertise. Training should cover not only the technical aspects but also an understanding of the domain in which they are working. For instance, annotators working for a locksmith service should understand various key types and security systems.
Step 4: Review and Quality Assurance
Implementing a robust review process is vital to maintain high-quality annotations. This may include peer review systems or the use of automated quality checks to ensure that annotations meet the set standards.
Step 5: Continuous Improvement
Machine learning models are not static; they evolve over time. As models are updated, so should the data annotation practices. Continuous feedback loops should be established to refine guidelines and improve the quality of data annotation.
Challenges in Data Annotation
Despite its importance, data annotation has its challenges, including:
- Time-Consuming: Depending on the volume of data, annotation can be a lengthy process, especially if undertaken manually.
- Quality Control: Ensuring annotation quality can be intricate, particularly when different annotators are involved.
- Cost: Depending on the approach, costs can escalate, especially if hiring skilled annotators or investing in annotation tools.
- Adequacy of Data: A lack of diverse data can hinder the effectiveness of machine learning models, leading to biases if not properly annotated.
The Impact of Data Annotation on Industries
Data annotation holds immense significance across various industries, allowing for innovative applications and driving business efficiency.
Healthcare
In the healthcare sector, data annotation is used in radiology to label imaging data for disease detection, significantly improving diagnostic speed and accuracy.
Automotive
Autonomous vehicles rely on data annotation to process visual information from the environment, such as identifying pedestrians and other vehicles, which is crucial for safety.
Retail
In retail, sentiment analysis through text annotation helps businesses understand customer feelings regarding their products, allowing for better inventory and marketing strategies.
Security
Data annotation is used in security to enhance threat detection systems by classifying potentially harmful activities, thereby improving overall safety and response times.
How KeyMakr Leverages Data Annotation
KeyMakr, a prominent name in the realm of Home Services, Keys & Locksmiths, effectively utilizes data annotation to enhance its service offerings. Here’s how:
1. Customer Assistance
By implementing NLP techniques through text annotation, KeyMakr can analyze customer queries and feedback accurately. This capability allows them to provide quicker and more tailored responses to customer needs.
2. Predictive Maintenance
The company uses data annotation to label service records and customer interactions. By analyzing this data, they can predict when a customer may require locksmith services, allowing for proactive customer engagement.
3. Marketing Strategies
Data annotation helps in categorizing customer data, which aids in devising targeted marketing campaigns tailored to specific customer segments, leading to increased conversion rates.
The Future of Data Annotation in Machine Learning
As machine learning continues to evolve, so too will data annotation practices. The rise of automation, AI-assisted annotation tools, and crowdsourcing techniques are enhancing the efficiency and scalability of data annotation processes.
1. Automation
Automated annotation tools can significantly reduce the time required for data labeling, enabling businesses to focus on refining their machine learning models rather than getting bogged down in manual processes.
2. AI and Machine Learning in Annotation
Machine learning algorithms are being developed to assist in the annotation process itself, recommending labels based on previously annotated data and minimizing human error.
3. Crowdsourcing
Engaging the crowd for data annotation can help businesses reduce costs and time efficiently. Platforms that connect annotators with data projects can provide scalability when handling large datasets.
Conclusion
In conclusion, the significance of data annotation machine learning cannot be overstated. For businesses in the Home Services, Keys & Locksmiths sectors, effective annotation processes serve as the foundation for intelligent machine learning applications that enhance service quality and customer satisfaction. By embracing innovative data annotation strategies, companies can not only improve their operational efficiencies but also stay ahead of the competition in an increasingly data-driven marketplace.
As technologies continue to advance, the future of data annotation in machine learning looks promising, paving the way for smarter, more responsive businesses. Companies like KeyMakr are already taking the lead in utilizing these practices to transform their service delivery and position themselves as leaders in their respective fields.