Unlocking the Power of Image Annotation Tools for Machine Learning
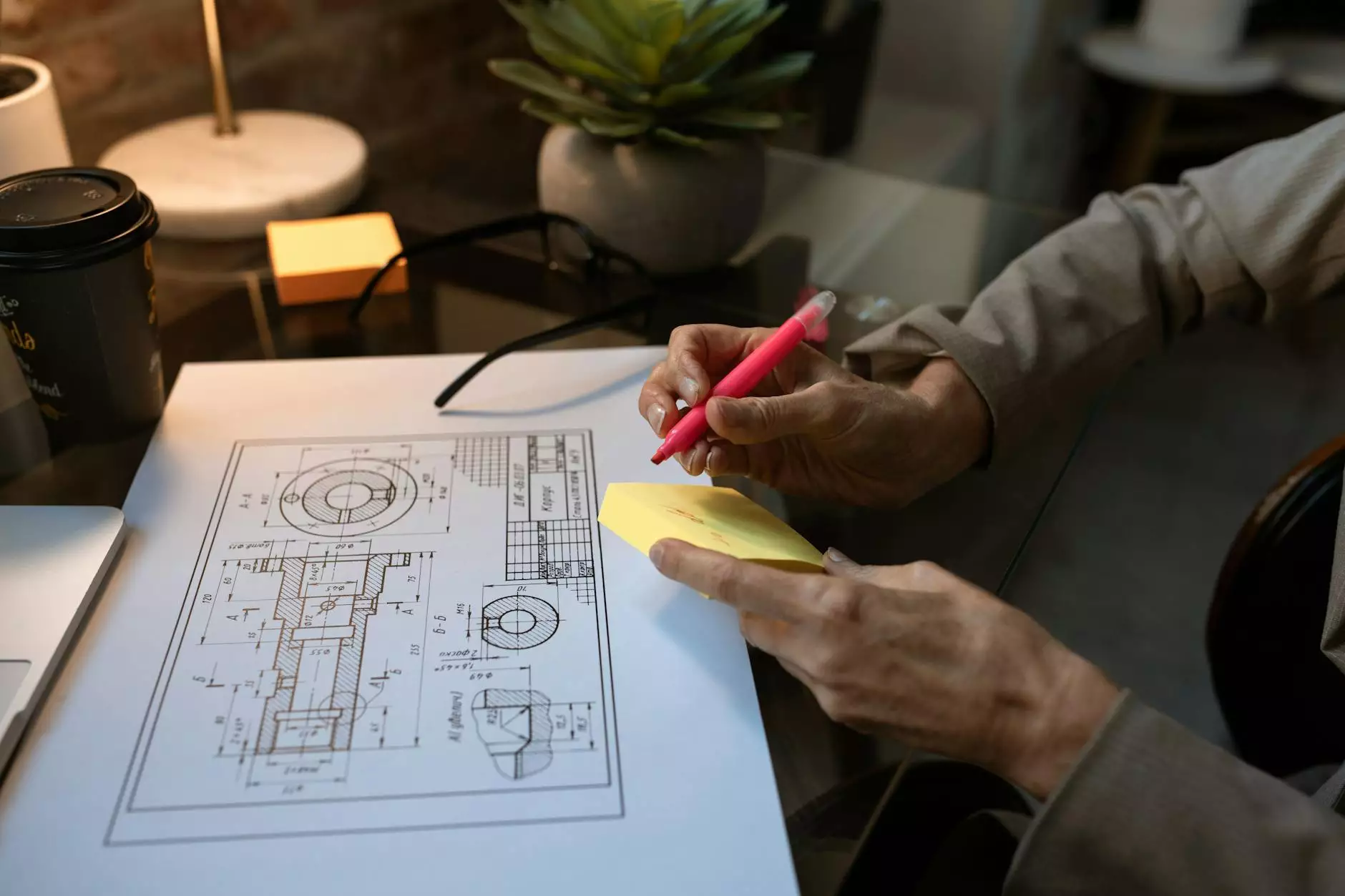
In recent years, the rise of artificial intelligence (AI) and machine learning (ML) has transformed industries, creating a demand for advanced data processing techniques. One of the critical components that influence the efficacy of AI and ML models is the quality of the training data. Specifically, image annotation tools for machine learning play a crucial role in enhancing data through annotation, enabling a better understanding of images across various applications.
The Importance of Image Annotation in Machine Learning
Image annotation refers to the process of labeling images with descriptive tags or categories, which enables machines to understand and interpret visual data. This process is essential for a variety of reasons:
- Improved Model Accuracy: High-quality annotations help machine learning models make better predictions by providing a clear understanding of what the data represents.
- Enhanced Data Quality: Accurate annotations lead to cleaner datasets, reducing noise and improving the model's learning capabilities.
- Facilitating Supervised Learning: Most machine learning models require labeled data for training, making annotation a foundational step in developing effective algorithms.
- Diverse Applications: Image annotation has applications in various fields, including healthcare, security, retail, and autonomous driving, highlighting the importance of adaptable annotation tools.
Exploring Image Annotation Tools
When it comes to choosing the right image annotation tool for machine learning, it’s important to consider several features that can greatly affect the workflow and outcomes of your projects. Here are some of the essential elements to look for:
1. User-Friendly Interface
A user-friendly interface is crucial for ensuring that both technical and non-technical users can operate the annotation tool effectively. A clean, intuitive layout minimizes the learning curve and allows for efficient annotation workflow.
2. Versatile Annotation Formats
Different projects may require various annotation types such as bounding boxes, polygons, or segmentation masks. A robust image annotation tool should support multiple formats to cater to diverse project needs.
3. Collaboration Features
In many scenarios, image annotation is a team effort. Tools that enable collaboration through shared projects, version control, and real-time editing options can greatly enhance productivity.
4. Integration Capabilities
It’s essential that the image annotation tool integrates seamlessly with your existing data pipelines, machine learning frameworks, and cloud services. This facilitates smoother data handling and reduces manual overhead.
5. Automation and AI Assistance
Some advanced annotation tools incorporate AI and machine learning features to automate parts of the annotation process. This can drastically reduce the time and effort spent on labeling images, allowing teams to focus on more complex tasks.
Keylabs.AI: Redefining Data Annotation with Cutting-Edge Technology
Keylabs.AI offers an innovative platform tailored for businesses seeking effective image annotation tools for machine learning. With a robust suite of features and an emphasis on quality and efficiency, Keylabs.AI is revolutionizing the data annotation landscape.
Features of Keylabs.AI's Image Annotation Tool
Keylabs.AI’s image annotation platform boasts several standout features:
- Customizable Workflows: Businesses can tailor the annotation workflow to fit their specific needs, enabling a more efficient data processing pipeline.
- Highly Accurate Annotations: Leveraging machine learning algorithms, Keylabs.AI ensures that annotations are not only fast but also precise, improving dataset quality.
- Data Security: Keylabs.AI prioritizes the security of your data, employing encryption and stringent access controls to protect sensitive information.
- Scalability: The platform can easily scale to accommodate large volumes of images, making it suitable for projects of any size.
- Real-Time Collaboration: Teams can collaborate in real-time, allowing for feedback and refinement of annotations as projects progress.
The Role of Data Annotation Platforms in the AI Ecosystem
The increasing reliance on AI in various sectors necessitates efficient data annotation platforms that can manage, annotate, and deliver data promptly. Here are some key roles these platforms play in the AI ecosystem:
1. Streamlined Data Preparation
Data annotation platforms simplify the process of preparing datasets by providing tools that make annotation quick and accurate, ensuring that models receive high-quality input for better training outcomes.
2. Facilitating Iteration and Improvement
With features such as feedback loops and performance tracking, businesses can continually refine their models based on annotated data, allowing for ongoing improvements in AI solutions.
3. Supporting Diverse AI Applications
From computer vision to natural language processing, quality annotated data is critical. Annotation platforms prepare datasets that support a wide range of AI applications, driving innovation across industries.
Best Practices for Image Annotation Projects
To achieve optimal results with your image annotation projects, consider these best practices:
1. Define Clear Guidelines
Establish clear annotation instructions to ensure consistency across the team. Guidelines should define what constitutes accurate labeling and address any specific challenges that may arise.
2. Utilize Quality Checkpoints
Implement quality control measures such as peer reviews or automatic checks to maintain high standards throughout the annotation process.
3. Invest in Training
Provide thorough training for your team members to ensure they understand both the tools and the specific requirements of the project. Investing in training pays off with better annotations and higher-quality datasets.
4. Monitor and Adjust
Regularly monitor the progress of the annotation project. Promptly address any issues that arise and adjust workflows as necessary to maintain efficiency and accuracy.
Conclusion
Leveraging image annotation tools for machine learning has become a pivotal part of developing robust AI applications. By utilizing advanced platforms like Keylabs.AI, businesses can ensure they are harnessing high-quality, accurately annotated data, thus paving the way for successful machine learning implementations and fostering innovation across various sectors. In a world defined by data, investing in the right image annotation tools is not just beneficial; it is essential for staying competitive in the rapidly evolving landscape of AI and machine learning.
For businesses looking to enhance their data annotation processes, exploring the offerings of Keylabs.AI can lead to transformative results. Discover how their solutions can elevate your machine learning workflows today.